5
1
9780471495178
Recurrent Neural Networks for Prediction: Learning Algorithms, Architectures and Stability / Edition 1 available in Hardcover
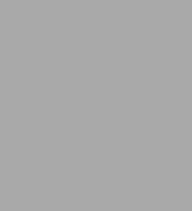
Recurrent Neural Networks for Prediction: Learning Algorithms, Architectures and Stability / Edition 1
- ISBN-10:
- 0471495174
- ISBN-13:
- 9780471495178
- Pub. Date:
- 09/05/2001
- Publisher:
- Wiley
274.95
In Stock
Product Details
ISBN-13: | 9780471495178 |
---|---|
Publisher: | Wiley |
Publication date: | 09/05/2001 |
Series: | Adaptive and Cognitive Dynamic Systems: Signal Processing, Learning, Communications and Control |
Pages: | 304 |
Product dimensions: | 6.83(w) x 9.72(h) x 0.90(d) |
About the Author
From the B&N Reads Blog