5
1
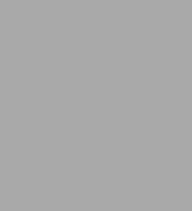
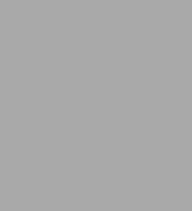
Paperback
$29.95
-
PICK UP IN STORECheck Availability at Nearby Stores
Available within 2 business hours
Related collections and offers
29.95
In Stock
Overview
Important text examines most significant algorithms for optimizing large systems and clarifying relations between optimization procedures. Much data appear as charts and graphs and will be highly valuable to readers in selecting a method and estimating computer time and cost in problem-solving. Initial chapter on linear and nonlinear programming presents all necessary background for subjects covered in rest of book. Second chapter illustrates how large-scale mathematical programs arise from real-world problems. Appendixes. List of Symbols.
Product Details
ISBN-13: | 9780486419992 |
---|---|
Publisher: | Dover Publications |
Publication date: | 12/28/2011 |
Series: | Dover Books on Mathematics Series |
Pages: | 560 |
Sales rank: | 1,168,718 |
Product dimensions: | 5.50(w) x 8.50(h) x (d) |
Table of Contents
1. Linear and Nonlinear Programming1.1 Unconstrained Minimization
1.2 Linear Programming
1.2.1 Simplex Method
1.2.2 Revised Simplex Method
1.2.3 Duality in Linear Programming
1.2.4 Dual Simplex and Primal-Dual Algorithms
1.3 Nonlinear Programming
1.3.1 Convexity
1.3.2 Kuhn-Tucker Conditions
1.3.3 Saddle Points and Sufficiency Conditions
1.3.4 Methods of Nonlinear Programming
References
2. Large Mathematical Programs with Special Structure
2.1 Introduction
2.2 Activity analysis
2.3 Production and Inventory Problem
2.4 Dynamic Leontief Model
2.5 Angular and Dual-Angular Structures
2.6 Linear Programs with Many Rows or Columns
2.7 Nonlinear Programs with Coupling Variables
2.8 Mixed-Variable Programs and a Location Problem
Problems, References
3. The Dantzig-Wolfe Decomposition Principle
3.1 Introduction
3.2 A Theorem on Convex Combinations
3.3 Column Generation
3.4 Development of the Decomposition Principle
3.5 Example of the Decomposition Principle
3.6 Economic Interpretation of the Decomposition Principle
3.7 Lower Bound for the Minimal Cost
3.8 Application to Transportation Problems
3.9 Generalized Transportation Problems and a Forestry-Cutting Example
3.10 Optimal Allocation of Limited Resources
3.10.1 General formulation
3.10.2 Specializing the Model--Lot Sizes and Labor Allocations
3.10.3 Computational Experience
3.11 Primal-Dual Approach to the Master Program
3.11.1 Linear Fractional Programming
3.11.2 Application of the Primal-Dual Method to the Master Program
3.11.3 Example of the Primal-Dual Method
3.12 Three Algorithms for Solving the Master Program--A comparison
Problems, References
4. Solution of Linear Programs with Many Columns by Column-Generation Procedures
4.1 The Cutting-Stock Problem
4.2 Column-Generation and Multi-item Scheduling
4.3 Generalized Linear Programming
4.4 Grid Linearization and Nonlinear Programming
4.4.1 General Development
4.4.2 Nonlinear Version of the Dantzig-Wolfe Decomposition Principle
4.5 Design of Multiterminal Flow Networks
Problems, References
5. Partitioning and Relaxation Procedures in Linear Programming
5.1 Introduction
5.2 Relaxation
5.3 Problems with Coupling constraints and Coupling Variables
5.4 Rosen's Partitioning Procedure for Angular and Dual-Angular Problems
5.4.1 Development of the Algorithm
5.4.2 Computational Considerations
5.4.3 Computational Experience
5.4.4 Example of Rosen's Partitioning Method
Problems, References
6. Compact Inverse Methods
6.1 Introduction
6.2 Revised Simplex Method with Inverse in Product Form
6.3 Upper Bounding Methods
6.4 Generalized Upper Bounding
6.4.1 Development of the Algorithm
6.4.2 Example of the Generalized Upper Bounding Method
6.5 Extension to Angular Structures
Problems, References
7. Partitioning Procedures in Nonlinear Programming
7.1 Introduction
7.2 Rosen's Partitioning Algorithm for Nonlinear Programs
7.2.1 Development of the Algorithm
7.2.2 Use of Partition Programming in Refinery Optimization
7.3 Benders' Partitioning Algorithm for Mixed-Variable Programming Problems
7.3.1 Development of the Algorithm
7.3.2 Relation to the Decomposition Principle and Cutting-Plane Algorithms
7.3.3 Application to a Warehouse Location Problem
7.3.4 Numerical Example
7.3.5 Computational Experience
Problems, References
8. Duality and Decomposition in Mathematical Programming
8.1 Introduction
8.2 Decomposition Using a Pricing Mechanism
8.3 Saddle Points of Lagrangian Functions
8.3.1 Basic Theorems
8.3.2 Everetts Theorem
8.3.3 Application to Linear Integer Programs
8.4 Minimax Dual Problem
8.5 Differentiability of the Dual Objective Function
8.6 Computational Methods for Solving the Dual
8.7 Special Results for Convex Problems
8.8 Applications
8.8.1 Problems Involving Coupled Subsystems
8.8.2 Example--Optimal Control of Discrete-Time Dynamic Systems
8.8.3 Problems in Which the Constraint Set is Finite: Multi-item Scheduling Problems
Problems, References
9. Decomposition by Right-Hand-Side Allocation
9.1 Introduction
9.2 Problem Formulation
9.3 Feasible-Directions Algorithm for the Master Program
9.4 Alternative Approach to the Direction-Finding Problem
9.5 Tangential Approximation
Problems, References
Appendix 1. Convex Functions and Their Conjugates
Appendix 2. Subgradients and Directional Derivatives of Convex
References; List of Symbols; Index
From the B&N Reads Blog
Page 1 of